#12 - Forging fit between data and business to win as data leaders
And how to make decision, tell great story, and understand culture with data
This Week’s Principle
Key Elements of the Decision-Making Process - Simplified
Opening the newsletter with what I call “idea-translation”, I’ll translate Peter Drucker’s brilliant but complex ideas into simple layman terms. Here are the five key elements of Peter Drucker’s decision-making process, simplified [1]:
“The clear realisation that the problem was generic and could only be solved through a decision which establishes a rule, a principle” => Use principles to solve problems, don’t be a pragmatist.
“The definition of the specifications which the answer to the problem had to satisfy, that is, of the “boundary conditions” => Set a clear and realistics definition of success.
“The thinking through what is right, that is, the solution which will the fully satisfy the specifications before attention is given to the compromises, adaptations, and concessions needed to make the decision acceptable” => Identify what is required to achieve the full “definition of success”, without factoring in compromises.
“The building into the decision of the action to carry it out” => Take actions; do what is required.
“The feedback which tests the validity and effectiveness of the decision against the actual course of events” => Observe reality’s feedback and reevaluate the decision.
--
[1] Summarised from The Effective Executive, Chapter 6, The Elements of Decision-making
This Week’s Strategy
Forging fit between data and business activities (link)
“Strategy is creating fit among a company’s activities. The success of a strategy depends on doing many things well - not just a few - and integrating among them.” -Michael E.Porter
While that is a principle for general strategists, it also has great applicability on data strategy. First, let us be clear that there are three levels of fit:
Simple consistency: This is where data activities align with business strategy.
Activities are reinforcing: One good activity makes the other better, and vice versa.
Optimisation of effort: Eliminate redundancy and minimise wasted effort.
For data leaders, the concept of creating fit between data and business activities deserves more attention. Here are some raw examples:
Simple consistency: Focus data efforts on cost analytics if the business strategy emphasises resource optimisation.
Activities are reinforcing: High-quality customer app usage data leads to improved features and better app engagement, resulting in even more data collected on customer’s activities.
Optimisation of effort: Data informs business about most important areas to focus on, while business indicates to data the most valuable data sources to acquire and maintain.
Forming a strategy that achieves activity-fit makes the organisation’s competitive advantage more sustainable. It also creates pressure for (data) operational effectiveness, as an ineffective area can have a ripple effect on the rest of the business operations. It’s a brave and brilliant way for data leaders to lead.
This Week’s Operation
Using the Stakeholder-Savvy Quadrant to win in data storytelling
To succeed in data storytelling, you first need to know your audience. How to do that? Lea Pica - a seasoned data analytics practitioner turned Data Storytelling trainer - introduced a framework called Stakeholder-Savvy Quadrant. This framework categorises your audience into four main personality types based on their "savviness" in two areas: business expertise and technical/data competence.
Here are the profiles of each quadrant and how to tailor data storytelling styles for each of them [2]:
Quadrant 1: High Business Expertise, Low Technical Savvy
People in this quadrant are the primary decision makers (C-suite, VPs). They care about their business goals, so you should precisely align your insights and messages with those goals. Use simple language to explain complex concepts, so you don’t lose the audience.
Quadrant 2: Low Business Expertise, Low Technical Savvy
This quadrant includes people in supporting functions (HR, quality control, etc), who assist in implementing the recommendation. In this case, focus on how they can help you get things done, rather than explaining the "why" of your analysis.
Quadrant 3: Low Business Expertise, High Technical Savvy
People in quadrant 3 are gatekeepers of the systems and processes, on which changes are to be introduced. They can be mid-level folks from IT or finance departments. They tend to be more risk-averse and under a lot of pressure to ensure all systems are ready, so they will scrutinise your findings carefully. As Lea said, “The best thing to do is to triple check your calculation and prepare for a questioning match.”.
Quadrant 4: High Business Expertise, High Technical Savvy.
This quadrant contains the toughest customers for you, who are possibly decision-making roles. The tip is to focus on the ultimate goal, which is the adoption of your idea. Avoid getting bogged down in the technical details if you can, and focus instead on gaining buy-in for your recommendation.
Being new to data storytelling, I find this framework neat, logical and helpful. I also think any good data storyteller should already be in Quadrant 4 - Do you agree?
--
[2] Content is transcribed and summarised from Lea Pica and DataCamp’s podcast here.
This Week’s Impact
Walt Hickey wants to track your eyeballs and make sense of culture (link)
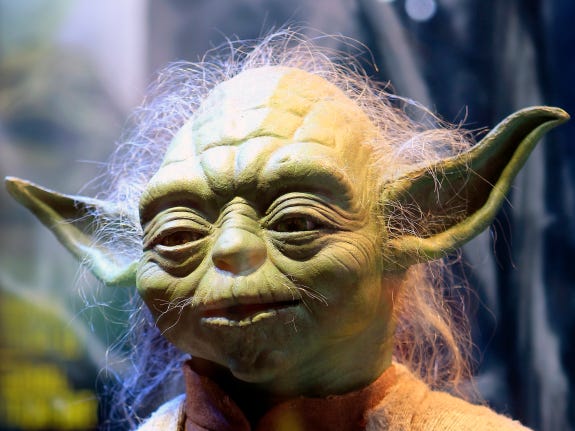
Understanding and making sense of culture is a difficult task. I often see it the task of critics, artists or journalists, who intuitively can generalise a string of events into a cultural phenomenon.
Not according to Walt Hickey - a data journalist who uses data to understand how culture works. I recently came across this Freakonomics’ podcast episode where he talked about how eyeball-tracking technology can help discover the reason behind a movie's popularity.
Using eyeball tracking technology, Walt Hickey studied where people look while watching the five Star Wars movies. For the first two movies, most people looked at the same spots at the same scene. While for the last three movies, people tended to look at different places for the same scene. Noticeably, the ratings for the first two movies are higher than the last three.
Walt Hickey saw this correlation a result of the director's effect. In the first two movies, the director had shot the scene in a way that focused the audience’s attention in exactly the spots he wanted. This brought out more of the important details in the story, and made the movie’s narrative clearer. In the last three movies, the director was different, and less skilled in capturing the audience's focus. The result is the audience were less clear on what the movie was trying to tell them.
Walt Hickey, not surprisingly a former scientist, had shown us that data can be gathered from many different places, using different forms of technology, and be applied to solve all kinds of problems, even the seemingly unquantifiable ones.
P/S: I also know of a great philosopher who can make sense of culture. He didn’t use statistical analysis, yet his use of observational data to infer cultural patterns are appropriately selective, logical and conceptual. Check out The DIM Hypothesis by Leonard Peikoff - one of the best books I have ever read in my life.
That’s it for this week! If you enjoy or get puzzled by the content, please leave a comment so we can continue the discussion. Throw in a like as or share as well if you know of someone who may enjoy this newsletter. Thanks!